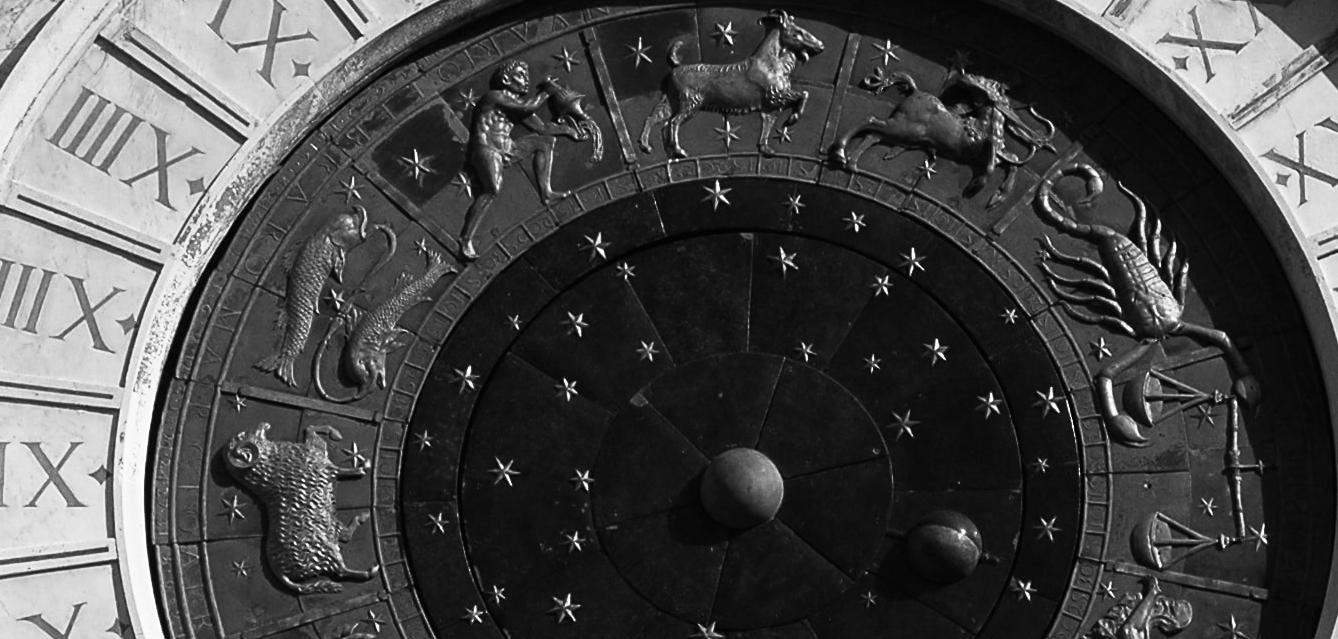
The Power of Asking "Why?" in Forecasting
June 16, 2022
“Data are profoundly dumb. Data can tell you that the people who took a medicine recovered faster than people who did not take it, but they can't tell you why. Maybe they took the medicine because they could afford it, and they would have recovered just as fast without it.”
Judea Pearl, “The Book of Why” intro
We've had a couple of exposures to COVID-19 at Well Principled. Even though multiple people were exposed at the same time, some contracted the virus confirmed by testing and a couple of fortunate teammates continue to test negative and have never exhibited any symptoms. They both happen to have O blood types, severe allergies, suffer from eczema, and are Pisces. I know we’ve all been wondering whether the combination of a Pisces Zodiac sign and O blood type indicates a natural immunity to COVID-19, but we also know that in the absence of results from a randomized control trial (RCT) or proven causal link, we would not be able to say whether water signs are, in fact, less susceptible to catching COVID.
The same concept applies in any kind of decision making process. A statistical model may make highly accurate predictions (at times), but it won’t tell you what might happen if something outside of the bounds of previously observed data is likely to happen. An example of this would be asking the question: “would our colleagues have gotten COVID if they weren’t in fact Pisces?”
In the world of consumer goods, forecast accuracy is crucial to meeting production targets, but if it doesn’t also inform the changes you could make - or could have made - in order to make your business more profitable, you might as well just use last year’s forecast. One step further, if you could give up a little (fake) “accuracy” for more action-ability, perhaps you should do that, because that kind of forecast would give you the power to understand what changes you could make to have a better outcome.
Forecast actionability = the ability to correctly predict the impact of making a change to one or multiple inputs of the plan. Let’s see how this works by asking the following questions:
- If I don’t run this promo, will I be better or worse off in terms of gross profit?
- If I had Google Search spend in half, how much would sales have dropped?
- Given limited supply, where will I miss out on the least amount of sales under stock-out conditions? This is the problem many companies are facing, given the current climate
A highly accurate statistically-driven forecast will often fall apart when it comes to these kinds of questions, what the math world calls "interventions" and “counterfactuals”. In order to correctly capture counterfactuals, you must be able to model the cause and effect relationships at play, removing the effects coming from everything else that might be happening at the same time (exogenous causes).
Teams make these decisions all the time, usually based on gut feel or past experience, but most ML models can’t predict the impact of those kinds of changes. That’s why they’re called “black box A.I.”! However, the management science world has been teaming up with mathematicians and computer scientists to tackle these causal relationships for years. As professor Yoshua Bengio noted in a recent article in Wired,
“Deep learning needs to be fixed. It won’t realize its full potential, and won’t deliver a true AI revolution, until it can go beyond pattern recognition and learn more about cause and effect. In other words, deep learning needs to start asking why things happen.”
What Professor Bengio means by “pattern recognition” is that black box models are fundamentally associational: they discern and recognize patterns in the relationship of variables by modeling “business as usual.” When you remove one variable, the pattern is broken, and these models fall apart. A simple example: In business as usual, Christmas sales are usually high, and so is Christmas ad spend. An associational model can correctly tell you that sales are likely to be high again this Christmas. However, if you’re planning on making an intervention (such as dropping Christmas ads), you’ve broken the pattern of business as usual. These models can tell you nothing, because they only know the pattern of all associations, not that Christmas ads were causing Christmas sales, and without them, sales will be flat.
A casual model is different because academics work with RCT and other methods to determine the structure and shape of the cause-effect relationships, testing those effects and building models based on the proven results. In fact, to return to our potentially COVID immune Pisces on the team, an international research team is looking into it. And perhaps we will soon know if the cause is in our stars...or not.
Principled
Thinking
Crawl, Walk, Run is Antithetical to Transformation
Is "Crawl, Walk, Run" really the best approach to digital transformation?
Untangling correlation, causation and concurrency in order to build an effective strategy.
What’s Wrong With “Data-Driven Decisions”
Well Principled removes the ??? from data driven decision making with causal models
A New Approach to Managing Risk and Boosting Ad Spend ROI
Get more from your marketing dollars with Nucleus's ad spend optimization