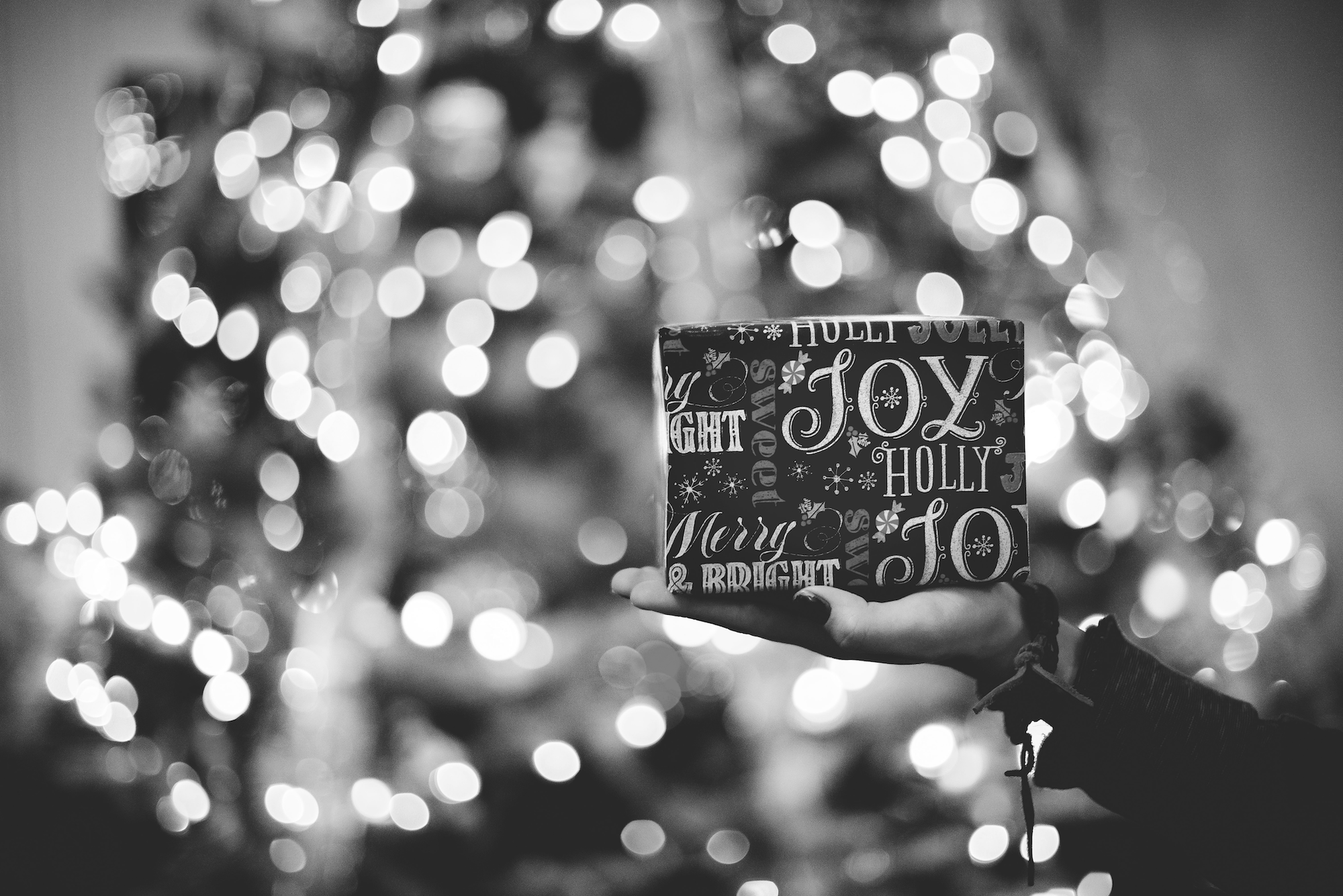
Be Right for the Right Reasons: Getting to Causality
June 16, 2022
It's not rocket science, right? You know that ad spend, promotions and seasonality affect your products' business performance. So why is it so hard to dial in changes to the factors that you can control and improve your outcomes?
Even if you are looking at dashboards and machine learning model results, you may be bumping into the limitations of navigating unknown waters with regards to your data. For example, many are familiar with the experience of last year's sales being an inaccurate performance indicator as we’ve ridden the wave of covid driven demand followed by a year of supply limitations. Or perhaps you are unwittingly impacted by limitations of directionality for your machine learning models where their predictive performance is predicated by irreversible mathematical dissection of the variables that are familiar into component aspects that you would not recognize. The arrow of time for decision making utility runs one way for this type of modeling approach, and they cannot be directly leveraged to answer questions about what to do when things change. A causal model where the relationship between variables is researched and defined is required to approach both prediction and intervention. The fine control you seek only occurs with a causal model, where you can not only intervene but then accurately predict based on that intervention.
The Three C's
In order to better understand how to put your data to work, let’s examine the three C’s- Correlation, Causation, and Concurrency, and how they impact your ability to use data to make data driven decisions. All three terms describe relationships between variables.
Correlation between variables indicates a relationship or tendency to occur together. Correlation might suggest a relationship but by definition it's only an unconfirmed association such as the famous relationship between ice cream sales and violent crime rates. Causation is when two variables are indeed confirmed to be related. This can be because one causes the other, like diet and diabetes, where there is an established and well-researched relationship. Correlation is not always causation but causation is always correlation.
Concurrency is when multiple variables or factors contribute to the occurrence of an event. In our diet and diabetes example, there are well documented other contributing factors like exercise and genetic predisposition. Diet, exercise and genetic predisposition are then concurrent causes or effects that result in the occurrence of diabetes. Since nothing exists in isolation, concurrent causes are inherent to causality. The same is true for the effect of ad spend, promotions, and seasonality on sales response that typifies the experience of many CPGs on Black Friday.
This issue of concurrency is why understanding causality is so hard. After all, no one is going to recommend to their leadership team that they do an experiment to discover this relationship between ad spend, promotions, seasonality, and sales response by not running ads and promos on Black Friday at all for the sake of discovering the impact of seasonality. That would be crazy!
Muddling Correlation and Causation
As described above, machine learning models are not built on causal relationships. This won’t stop providers of those solutions from trying to assign cause. How do you know if the solution that you are using is from machine learning or a causal model? Of course you should ask, but any of these should tip you off in the meantime:
- The discussion on causes centers around the measurement of correlation
- Some of the variables are surprising, for example a relationship between the price of coal and chocolate sales as shared in a true story by a major CPG regarding their Machine Learning vendor.
- The solution sales team advertises that the solution picks the best model (this is a tip off that the structure is unknown.)
- Engineers are talking about feature extraction
Leveraging Causal Models
Why is it important to ask? Effective interventions are based on causal models that can't just predict well, but also have to be right for the right reasons, or else the predictions under intervention will be wrong. This is especially critical when the economic environment is going through a high rate of change. Establishing a causal model framework requires some really hard research and is overwhelming if you considered taking on the task from scratch. The good news is that qualified researchers at the finest business schools have already taken on some of that heavy lifting. Well Principled leverages those peer reviewed published research papers to establish causal model frameworks which can operate well for prediction as well as intervention.
Why doesn’t everyone do that? The adaptation to a software solution that allows you to accurately dial in specific temporal changes in business strategy simultaneously requires deep mathematical understanding, software engineering skill, and business acumen. A high performing team with such combined expertise is not easily assembled, and even if they were, it would take years to catch up.
While it’s not rocket science, the science of establishing causal relationships is critical to an effective solution. With the causal model mastered on your behalf, you can effectively optimize ad spend and promotions for your products for every week of the year. When you have those “what if” questions, you can quickly respond and demonstrate why.
Principled
Thinking
Crawl, Walk, Run is Antithetical to Transformation
Is "Crawl, Walk, Run" really the best approach to digital transformation?
Exploring the limitations of common A.I. modeling approaches as applied to decision making
What’s Wrong With “Data-Driven Decisions”
Well Principled removes the ??? from data driven decision making with causal models
A New Approach to Managing Risk and Boosting Ad Spend ROI
Get more from your marketing dollars with Nucleus's ad spend optimization